If one sector benefits from adopting a data-driven approach, it’s software as a service (SaaS), particularly in a B2B context. The entire model of developing, marketing, and selling software on subscription is guided by data, or ought to be.
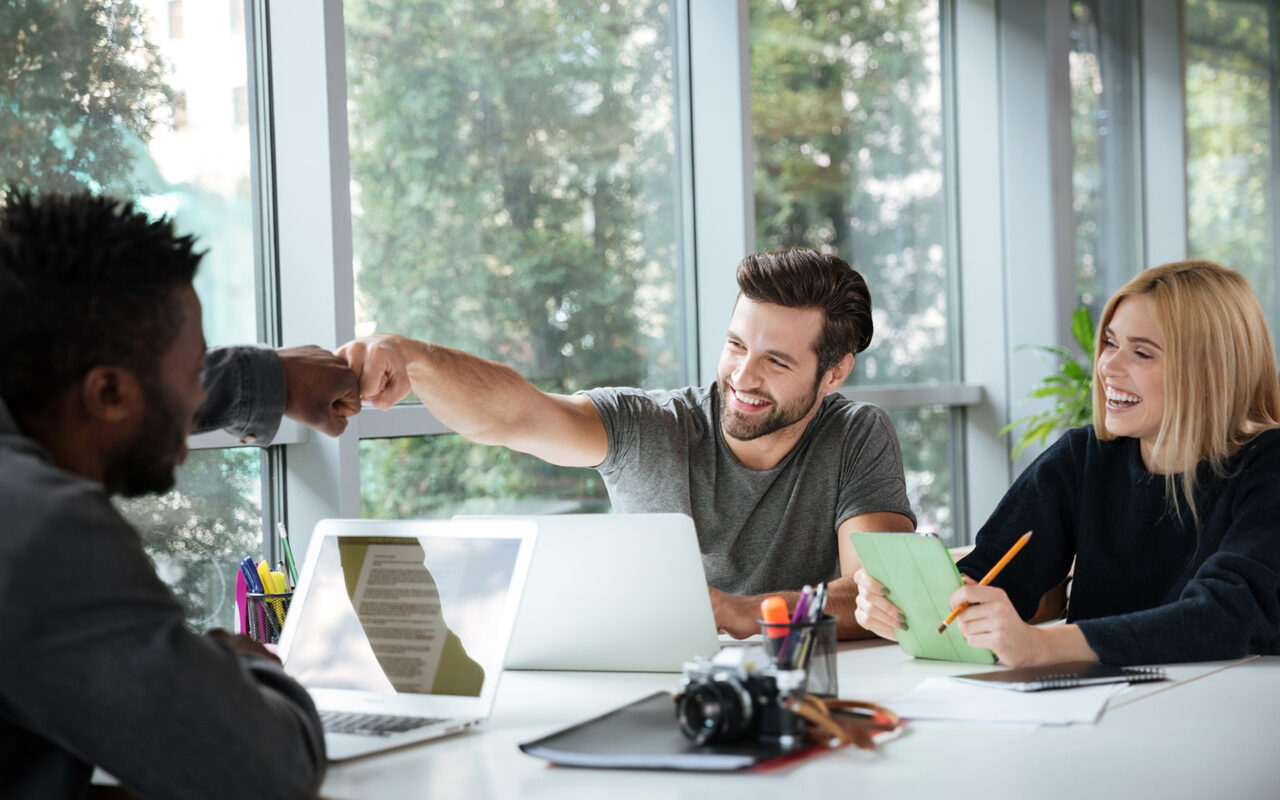
Developing a data-driven culture means you’re not relying on gut instinct. Or hearsay to find out what’s working or not about your marketing and sales strategies.
We’ll explore why it’s not only important to leverage big data to develop better products, find new markets, and reduce customer churn, but it’s essential.
Table of contents:
The Essence of a Data-Driven Culture
Key Components of Cultivating a Data-Driven Culture
Challenges in Building a Data-Driven Culture
Practical Steps to Implement a Data-Driven Culture
Case Studies: Real-World Examples of SaaS Companies Embracing a Data-Driven Mindset
The Essence of a Data-Driven Culture
What do we mean by “data-driven culture?”
We mean that the company makes its decisions by sourcing data, then identifying opportunities to improve things.
Data sources that might be accessed to fuel decision-making include:
- Customer usage data – how do customers typically use the product?
- Freemium to subscription conversion – do free trials translate into subs sales?
- User churn – how many customers renew, and how many do not?
- Product and feature uptake – which specific features are most popular?
- Competitor intelligence – what are your rivals doing?
- Customer sentiment analysis – what are customers saying on forums?
This non-exhaustive list gives a flavor of the data that can be accessed to support intelligent decision-making. In a data-driven culture, such data is sourced, maintained, and analyzed regularly to maintain a superior level of business intelligence.
Key Components of Cultivating a Data-Driven Culture
A data-driven culture isn’t only about data sourcing and analytics, however. The specific approach to data governance is important too.
Five key features of a data-driven culture include:
Data Accessibility and Quality
There’s no point in attempting to instill a data driven culture unless you have access to high quality data. Such data should be accurate, consistent (to pre-set rules), complete, valid (properly formatted), and up to date. It needs to be available at a scale sufficient to identify trends and project patterns.
Data Security and Privacy
It is important, in any data-driven workplace, to ensure data is securely stored, and that access privileges are ring-fenced. Further, regulations such as European and British GDPR and Californian CCPA privacy legislation should be followed.
Fortunately, many methods of securing data exist including state-of-the-art encryption, access control, and blockchain registration. To comply with privacy requirements, data can be aggregated and anonymized. This will ensure no individual’s information is accessed inappropriately.
Continuous Learning & Training
Central to a data-focused culture is fostering continuous learning and development in SaaS. Even employees without a data-centric role can use analytics, automation, and AI systems to optimize their roles.
From automatically sending subscription reminders to generating personalized marketing campaigns, AI and automation increase efficiency and productivity. Employees who continue to learn how such systems benefit them will flourish in a data-driven culture.
Data-Driven Decision Making
There’s no use in employing data analytics at scale, if you don’t make decisions based upon its findings. That doesn’t mean you can’t override a suggestion prompted by an analytic process, since pure creativity and innovation are often outside data science’s purview.
However, it makes sense to use customer behavioral data, market data, and competitive intelligence to drive intelligent decision-making. There’s a mountain of evidence that such decisions stand a better chance of being the right ones.
Data Sharing and Reporting
When data is siloed in different departments, it limits the insights a business can obtain. Customer service and support often have as much to offer as R&D and marketing.
Another vital aspect of the date-driven culture is data sharing and the circulation of trend reports based upon integrated data from different departments.
As well as improving morale and fostering inter-office collaboration, such an approach leads to better-informed decisions and more actionable insights.
Challenges in Building a Data-Driven Culture
Given the many advantages to creating a data-driven culture, what are the obstacles businesses face in adopting this approach?
Here are some obstacles:
- Lack of understanding: executives haven’t explained the strategy convincingly.
- Partial buy-in: some departments are more data-centric than others.
- Concerns over replacement: employees may worry about being replaced.
- Lack of planning: the GANTT chart for project management hasn’t been thought through.
- Insufficient budget: management hasn’t allocated sufficient resources.
- Learning and development inadequate: the learning curve is steep and poorly managed.
- Badly integrated tech stack: the wrong tools and platforms have been purchased.
Practical Steps to Implement a Data-Driven Culture
Given the above problems, what solutions might be implemented to counter these difficulties?
Here are some pointers:
Strategy and Vision: There should be clear communication from the top down about the importance of data and its role in decision-making. Leaders need to articulate their vision and align it with strategic objectives, ensuring everyone understands how their actions contribute to data-driven goals.
Employee Engagement and Training: Invest in comprehensive training programs to enhance data literacy across all departments. Ensure every team member, not just data scientists or analysts, understands how to use and interpret data.
Technology Integration: Evaluate and integrate technology that supports data-driven decision making. This may include CRM systems, data analytics platforms, and other tools that facilitate data collection, analysis, and visualization.
Data Quality Management: Establish robust data management practices to maintain high-quality, accurate, and timely data. This includes setting up systems for data cleansing, validation, and regular audits to ensure data integrity.
Creating Feedback Loops: Implement feedback mechanisms. They allow employees to share how data-driven changes are impacting their work. This not only promotes a sense of ownership but also helps identify areas for improvement.
Encourage Experimentation: Foster an environment where employees feel safe to experiment and learn from data-driven projects. This could involve setting up a sandbox environment where teams can test ideas without the risk of significant repercussions.
Case Studies: Real-World Examples of SaaS Companies Embracing a Data-Driven Mindset
The SaaS industry is growing fast and is expected to reach a market volume of $344 billion (about $1,100 per person in the US) by 2027. Here are just three examples of extraordinarily successful data-driven companies in the SaaS or subscription model sector:
Case Study 1: Activision
The leading games brand, Activision Blizzard, actively monitors social media conversations to gauge customer sentiment. This approach has proven effective, as evidenced by their impressive quarterly net revenue of $2.2 billion USD (approximately $7 per person in the US) in the second quarter of 2023.
Case Study 2: Blackbird.ai
This start-up has created an AI-driven anti-misinformation platform Constellation that is “capable of identifying and highlighting threat actors, entities of interest, accounts (including bots/botnets and/or influencers), coordinated communities, connections, photos, videos, and data streams.”
Blackbird’s blog demonstrates their commitment to keeping pace with AI and deepfake technology to offer their customers innovative solutions.
Case Study 3: Netflix
Without being able to leverage its immense reservoir of user behavior data, Netflix would not have become the profitable powerhouse in streaming and production it is today.
It uses this data to identify trends, curate personalized recommendations, and maximize time on the platform, thus building customer loyalty and generating revenue.
These case studies illustrate how a data-driven mindset leads to improvements in product development, customer satisfaction, and operational efficiency.